We offer the following expertise for healthcare business analytics:
- Cluster Analysis and Segmentation
- Enrollment & Churn Rate Analysis
- Forecasting
- KPIs Definition and Dashboards
- Customer Feedback Analysis
- Manufacturing Operations Optimization
Applications are targeted on healthcare products & services. Oehoe Data Science has domain expertise in the field of medical imaging, disposables and digital solutions.
With clustering techniques we on the one hand group data in segments wherein data attributes behave similar. On the other hand we set groups apart with data attributes behaving different. As such we group together what belongs together and we differentiate where appropriate. It takes fair judgement while optimizing the clustering and segmentation. There’s a trade-off between increased segmentation to foster a tailored proposition and keeping the segmentation level simple for efficiency reasons.
Enrollment & Churn Rate Analysis follows the lean analytics approach. The enrollment rate measures how many respondents start testing or using your product or service. The churn rate measures how many users leave in a given time period. Both parameters can be measured on historical data, yet thanks to the technique of survival analysis one can also predict the probability of a specific user to leave. This is valuable for targeting dedicated user groups for sustained engagement.
Value Assessment is a structured way to understand and frame value drivers. Value is often confused by excessive focus on product features. It is crucial to take the perspective from the user in understanding their experience with the offered solution, what drives their behavior and their willingness to purchase. Value drivers are identified, weighed and scored in consultation with a user test group. This can be compared with a competitor’s scoring, to the extent this information is available. Next, we turn value into money by using a Net Present Value model or equivalent. This allows us to create a value model of the offering at stake.
Data Visualization is meaningful to better understand data properties, to find patterns as a precursor for a modeling strategy, to debug your analysis and to convey your message to the user. Analytical graphics allow to compare results in a treatment/placebo study, to show correlations and causality where appropriate, to visualize multi-dimensional data and to annotate the evidence with legends and additional information where needed. A variety of plots are used: histograms, boxplots, barplots, scatter plots, cluster plots & dendrograms.
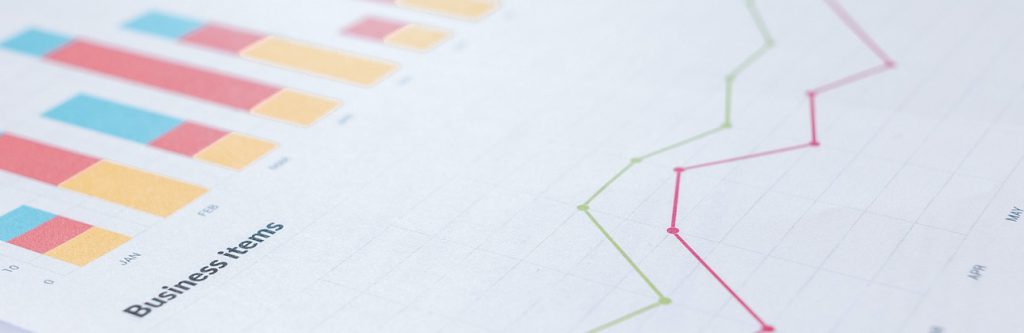